INTRODUCTION
The world of art authentication has long been a delicate dance between tradition and technology. Connoisseurs, curators, and experts have spent centuries honing their ability to identify and authenticate works of art. However, the advent of artificial intelligence (AI) has revolutionized the art authentication process, introducing unprecedented precision, objectivity, and speed. In this article, we will explore how AI is being used to identify and authenticate works of art, ultimately affecting museums, galleries, and collectors.
THE TECHNOLOGY BEHIND AI
To understand just how advantageous AI art authentication is, it is essential to delve into the inner workings of its technology. Initially, an AI system undergoes a training phase in which it is introduced to a collection of known works by a particular artist, often referred to as a catalogue raisonné. A key advantage to this approach is that the catalogue raisonné need not be physically present; the AI system can learn such attributes from digital renderings of paintings. The piece going through the authentication process also does not need to be in the same room as the AI system; a photograph of a painting taken on an iPhone is of sufficient quality to be properly AI-authenticated.
For the AI technology to function effectively, a minimum of 100 images is required. The system is also fed images forged in the style of a particular artist to allow it to discern an imitation from an authentic work. The system acquires an understanding of the artist’s technique and key characteristics from these reference images. This stage can take up to three days, depending on the complexity of the artist’s style.
The learned AI system next conducts a 3D scan of the painting using a chromatic confocal profilometer device. This new 3D topography technology is able to go beyond traditional methods of manual human art authentication that predominantly revolve around studying brushstroke shapes. Unlike less advanced AI authentication methods, 3D topography does not analyze the entire painting, but rather identifies the artist based on a select surface area of the painting. The device relies on light and color to formulate a highly detailed map that reveals the subtle textures within a painting. The system will then meticulously compare and analyze both the depressions and elevations of the painting’s surface and the microscopic patterns of the artist’s brush. The AI can also detect any fingerprints within the work that could not be seen with the human eye.
After this process is complete, the system delivers a class probability assessment to indicate whether the painting is likely the work of a specified artist. The AI sorts the image into one of the two classes of work it has learned: the authentic dataset or the contrast dataset, which contains only forged work. Because these AI authentications currently operate at around an 85% accuracy rate, they are frequently used in conjunction with human art historians to offer a reliable and comprehensive authenticity analysis.
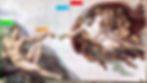
AI’S ROLE IN IDENTIFYING/ATTRIBUTING
Computers have long played a role in analyzing and categorizing artwork, but advancements in AI have added a new tool for curators and collectors alike. AI algorithms have been developed to analyze the digital representations of artwork with incredible precision. Artists often exhibit distinctive styles and techniques which evolve over time. AI can identify and trace these evolutions, helping in the dating and attribution of works. For instance, algorithms can detect if a work is more characteristic of an artist's early or later period, providing valuable insights for authentication. The machine learning models behind AI can identify minute details such as brushstroke patterns, color palettes, and even hidden layers beneath the visible surface.
One example of this technology at work was displayed in the attribution of The de Brécy Tondo. In 1981, collector George Lester Winward purchased the Tondo painting with full belief that the Italian Renaissance painter and architect was responsible; however, art galleries and museums believed it to be a copy of Raphael’s Sistine Madonna. Leading up to his death, Winward established a trust and donated his collection to be studied, with works in various mediums ranging from the sixteenth-century Italian schools and seventeenth-century Dutch and Flemish painters—The de Brécy Tondo being the most mysterious and controversial of the collection.
Previously, researchers sorted through some of the nebulous origins in 2007, narrowing the painting’s window of creation to prior to 1700. This newest discovery comes with aid from facial recognition technology and AI through learning human facial structures and identifying unique attributes of each work. Some of these features are unrecognizable to the human eye (think about when someone looks familiar, but you’re not sure how or from where); however, “these facial recognition systems can compare two facial images in much greater detail and can outperform humans,” says Hassan Ugail, a professor of visual computing at Bradford. Researchers used Raphael’s Sistine Madonna for the comparative image analysis, with the technology finding that the two Madonnas shared 97% of traits, and the two versions of baby Jesus have 86% similar characteristics. The potential for this technology is groundbreaking; however, it is worth mentioning that a different AI model has disputed the painting as the work of Raphael. It opens the door to potentially identifying other unattributed works to their creators and possibly identifying more detailed tenets of artistic movements and eras.
AI’s ROLE IN VERIFICATION/FORGERIES
AI has opened new horizons in the field of art authentication. Authentication is a complex process that involves scrutinizing various aspects of a work, from brushstroke style and materials used to historical context. Traditionally, this has been the domain of art experts, who, through years of study and experience, have developed an intuitive understanding of an artist’s unique characteristics. However, human judgment is not without its limitations, and the subjectivity inherent in the process often leads to disputes, errors, and forgeries slipping through the cracks. AI offers unparalleled precision, objectivity, and speed in scrutinizing artworks and identifying forgeries. An additional facet of this use case relates to provenance. Provenance is essentially the record of an artwork’s journey from origin through different hands of ownership, and this information is used to build context around the work. Most legal conflicts surrounding masterpieces involve disputes over ownership rights. For examples, see Howard University v. Borders & King v. Wang.
Part of the AI authentication process ensures that the provenance and value of art are preserved through ownership records and carefully scans the work by finding subtle inconsistencies down to the brushstrokes in order to identify forgeries. Commonly, art forgery is thought to entail an exact replica of a work. However, most forgeries are actually new works simply done “in the style” of famous artists. These forgeries compose a lucrative market for fraudulent actors, and an auction house or gallery selling forged works could be crippled if their works are discovered to be fakes. In a recent example of this, the Knoedler Gallery in New York had to shut down due to controversies surrounding the legitimacy of nearly forty of its auctioned works. This demonstrates the necessity of rigorous and careful authentication processes when receiving a work.
AI has been able to identify counterfeit artwork with a high success rate so far. One such example is Max Pechstein’s Seine Bridge with Freight Barges. In 2010, the infamous art forger, Wolfgang Beltracchi, was arrested by German authorities and accused of forging over thirty-six paintings for nearly $45 million. Art-Recognition, a Zurich-based firm that creates algorithms and data sets to authenticate works, classified the piece as “non-authentic” with 94.75% certainty. The same company has also identified London’s National Gallery as having a non-authentic Paul Rubens painting, Samson and Delilah.
CONCERNS
While the success stories of this technology are promising, the art community has cautiously approached this innovative process. Richard Polsky, an art authenticator focused on 20th-century American artists, has expressed as much by saying:
If you’re deeply immersed in an artist’s work, you’ve read everything about them. You’ve been to all the museums all over the world to see originals, you’ve been to gallery exhibitions, maybe you’ve owned a few or bought and sold them . . . . I don’t think that sort of thing can be taught.
Additionally, art classification networks have had some difficulty distinguishing between cityscapes and landscapes, only recognizing that both are outside. Some of these challenges have prompted art experts to view the technology with skepticism; however, the potential for implementation is likely to continue growing.
There are also hurdles in the legal landscape that make AI’s application difficult to predict. Specifically, the Federal Rules of Evidence are established and tested foundations for legal trials that, in many ways, prevent AI conclusions.
One one the first hurdles that AI authentication programs could face in court would be whether such evidence is considered hearsay. At first glance, it would seem inadmissible as it could be considered an out-of-court statement. The definition of hearsay goes as follows: “a statement that (1) the declarant does not make while testifying at the current trial or hearing, and (2) a party offers in evidence to prove the truth of the matter asserted in the statement.” If evidence of findings by an AI authentication software were presented in court, (1) it would be a statement not made while testifying at the current trial or hearing, and (2) it would be presented to show whether or not a painting is fake, which would be inadmissible hearsay. At least, this is the argument that was presented by the defendant in State v. Morrill. The court was asked to decide whether or not the work produced by AI tools (i.e., Roundup and Forensic Toolkit) constituted ‘statements.’
To answer this question, the court relied on the definition of hearsay mentioned above, along with the definition of declarant under the Federal Rules of Evidence. Rule 801 states that a declarant is defined as “the person who made the statement,” and a statement is defined as “a person's oral assertion, written assertion, or nonverbal conduct, if the person intended it as an assertion.” The district court admitted the evidence generated by Roundup, deeming it not hearsay as it originated from a computer rather than a human source. Similarly, the district court admitted the evidence produced by Forensic Toolkit, ruling it admissible under multiple grounds: either not being hearsay due to its computerized origin; or not offered for the truth of the matter asserted; or alternatively, if considered hearsay, its admission was supported by a sufficient foundation under the business record exception. The New Mexico Court of Appeals affirmed the lower court’s ruling on this matter, citing State v. Mendez: “The hearsay rule excludes from admissible evidence statements that are inherently untrustworthy because of the risk of misperception, failed memory, insincerity, ambiguity, and the like.” The Court of Appeals concluded that “statements produced by software are not subject to the same types of risks. Because the evidence produced by Roundup or Forensic Toolkit does not constitute hearsay, we conclude that the district court did not err in admitting the same.”
This same logic could be applied to AI art authentication software, and its findings could be offered as evidence to prove the authenticity of a work of art. However, this logic is not foolproof, as AI is a relatively new matter in the world of law. More tailored laws are expected to emerge as AI technology and its use grow more popular every day.
Another hurdle in the courtroom, besides what was said, is who said the statement. Expert witnesses, as opposed to lay witnesses, testify on the basis of their scientific, technical, or other specialized knowledge. Like those used for AI authentication, an abstract algorithm makes it difficult for jurors to assess credibility. Rule 702 sets forth four requirements: (1) the expert will help the trier of fact to understand the evidence or determine a fact in issue; (2) the testimony is based on sufficient facts or data; (3) the testimony is the product of reliable principles and methods; and (4) the expert has reliably applied the principles and methods to the facts of the case. While the algorithm will definitely help explain some of the evidence and is based on sufficient data, the latter two requirements are more challenging to overcome.
Data algorithms like AI are not necessarily new. However, their application to a wider range of use cases and fields of study is novel and may require some troubleshooting before being widely accepted. Also, the “reliable” application of these techniques to the facts of cases will prompt detractors to question whether these methods are consistently accurate. There are still questions as to how AI takes training data and arrives at the resulting conclusions, though, and any inconsistencies can affect a masterpiece’s value by millions, so the stakes are very high.
Furthermore, expert witnesses who have studied art, history, and artistic styles have a body of research and theses that are much more approachable than a computer’s output. As a result, juries will likely have a far easier time trusting an explained line of reasoning rather than a mathematical computation. That’s not to say there isn’t an application for AI in relation to expert witnesses. Recently, AI language models have been used to verify expert testimony for accuracy in depositions. In that case, software was used to scan testimony, totaling 7,500 pages across sixty-three depositions, to find points where a medical expert made inconsistent statements. AI was able to sift through these documents in forty-five minutes and found an error in a critical element in the case. Whether this technology will cross into the world of art authentication and recognition remains to be seen, however, the potential is there and certainly would create more efficient methods of vetting art historians and researchers alike.
Lastly, the introduction of AI as evidence presents a formidable challenge in navigating the complexities of admissibility determinations. Rule 104 allocates responsibility for determining preliminary questions of admissibility of evidence between the court and the jury. While the jury evaluates preliminary questions concerning evidence relevance, judges are entrusted with assessing the competency of evidence and the applicability of hearsay exceptions. The incorporation of sophisticated AI mechanisms into this process adds layers of complexity to determining admissibility. Judges, who are expected to possess expertise primarily in legal matters, may find themselves grappling with the technical intricacies of AI technology. Jurors, who are expected to function simply as laypeople, may struggle to comprehend and evaluate the relevancy of AI art authentications. Expecting judges and jurors to render legal determinations based on the complexities of AI stretches beyond their traditional functions in the court. This would threaten their ability to confidently answer preliminary questions of admissibility.
CONCLUSION
While challenges and debates surrounding AI’s role in the art world persist, one thing is clear—the fusion of human creativity and machine intelligence is opening new horizons for art, ensuring preservation and appreciation for generations to come. Ultimately, integrating artificial intelligence in art identification and authentication represents a transformative leap forward in the art world. The capabilities of AI, from image recognition algorithms to data analysis, have not only expedited the process of verifying the authenticity of artworks but have also revolutionized the way we approach art history and preservation. As this technology evolves and matures, it offers a promising future where the delicate dance of human expertise and AI-driven precision will collaboratively shape the art market.
*The views expressed in this article do not represent the views of Santa Clara University.